In the News
Special Edition on how data quality impacts safety and bias in AI
AI is playing an ever greater role in improving the safety of AI applications both in the digital and physical world. As with all aspects of machine learning, safety starts with the input quality of the data. Equally important is the need to focus on the diversity of situations the visual data adds to the machine’s ability to learn and predict outcomes. Rather than merely bad data, limited data has led to some examples of self driving cars not having the depth of learning to deal with driving situations in adverse weather conditions.
Trove, a high quality data marketplace from Microsoft, builds trusted connections between you and people who contribute to your projects – resulting in an ecosystem that fosters higher quality data and benefits everyone involved. With Trove, you can train AI models with data specific to your needs and Trust that data was responsibly sourced.
We take a look at three trends and examples. With COVID-19, the need to cultivate safe working spaces grew exponentially. Using anomaly detectors driven by computer vision, automatic monitoring was able to identify lapses or low compliance with precautions like mask wearing, PPE or social distancing.
Facial recognition technology is commonplace, used to access services and devices when privacy and identification are key needs. Recently Clear and Hertz Car Rental implemented a pick up system that allows customers to avoid the dreaded counter process and safely pick their vehicle simply by scanning their face with high compliance to protect the customer and the business in order to avoid fraudulent rentals or releasing personal information.
On a positive note, the AI fuelling self driving cars’ development seems to have learned from past mistakes. Volvo aims to equip the self-driving car with body language that everyone can understand, Mikael Ljung Aust from Volvo Cars Safety Centre says: "What we really need is three or four key sounds that tell you what the car is going to do. Sufficient computer vision around human motion is a critical success factor for this technology".
With Trove, you can train your AI models on images that are specific to your needs and improve the relevance of your data sets. You don’t have to worry about whether you are getting the best value for photos obtained and can trust that the data you collect will be done safely and responsibly through terms that respects the rights of submitters.
Sponsor
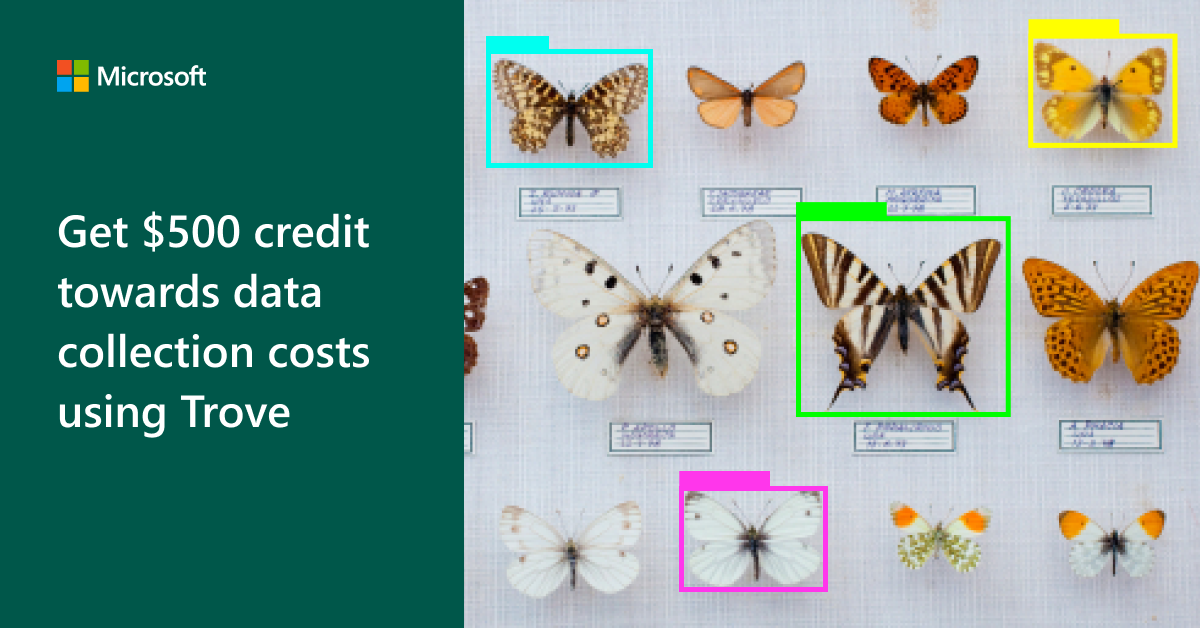
Get a $500 credit* towards image data collection costs using Trove
Trove is a new crowdsourcing marketplace from Microsoft where you can gather images for AI responsibly.
You get:
- Value: You don’t have to worry about whether you are getting the best value for photos obtained through Trove.
- Confidence: Data you collect will be done safely and responsibly through terms that respects the rights of submitters.
- Quality: Highly relevant and diverse images from real people, licensed for your specific scenario and use case.
*Eligibility for the $500 credit depends in part on having an acceptable Trove project and making payments via Trove. Full details on the offer and all eligibility requirements can be found in the official terms.
In The News
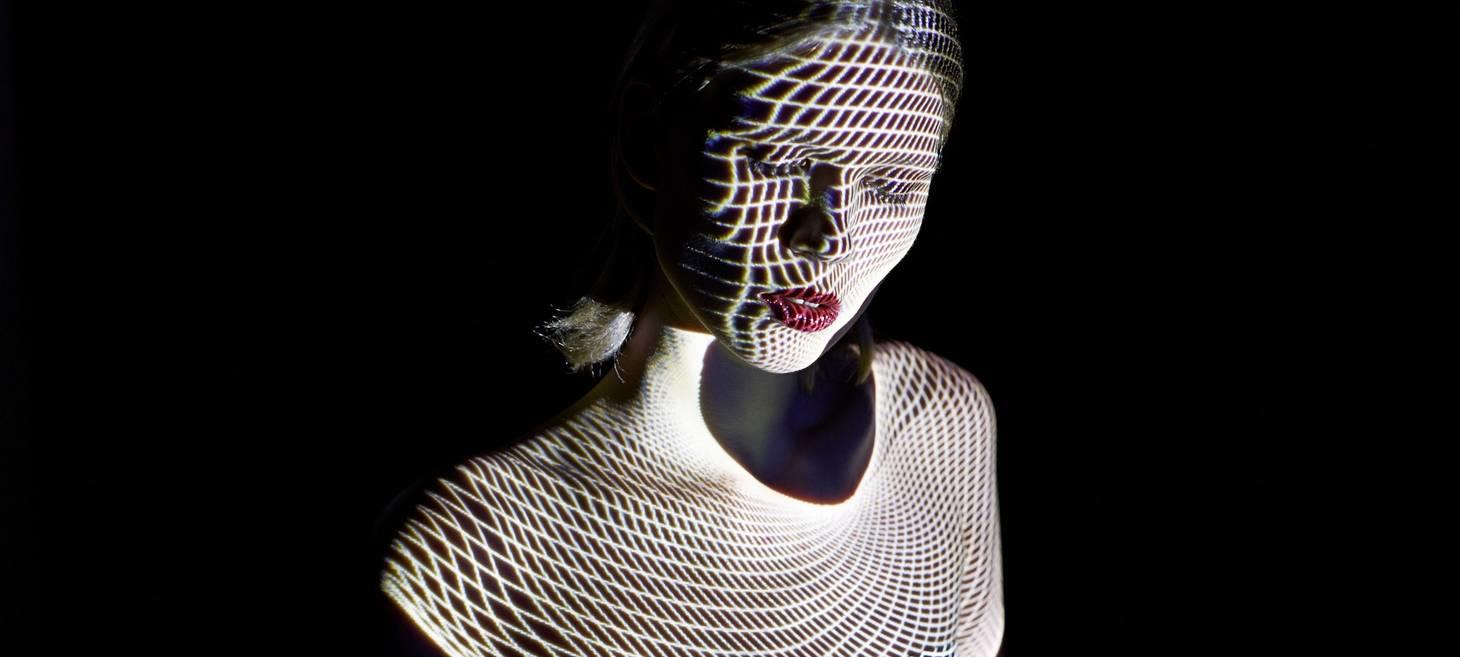
Researchers Blur Faces That Launched a Thousand Algorithms
IN 2012, ARTIFICIAL intelligence researchers engineered a big leap in computer vision thanks, in part, to an unusually large set of images—thousands of everyday objects, people, and scenes in photos that were scraped from the web and labeled by hand. That data set, known as ImageNet, is still used in thousands of AI research projects and experiments today.
A 5-step guide to scale responsible AI
Deploying AI at scale will be problematic until companies engage in fundamental change to become ‘Responsible AI’-driven organizations'
Applied use cases
Startup improves safety using deep learning-based computer vision.
The machine learning models are automatically re-trained as data is collected, so that the system's accuracy improves over time as it is used. Workers take before and after pictures of the job and real-time advice is provided, before the worker leaves the site.
There’s no going back: how AI is transforming recruitment
The accelerated use of artificial intelligence and machine learning by recruitment specialists over the past year is creating jobs by the thousand; it’s time for HR to fully embrace the new technology and work with it to avoid bias, argues AI expert Gez McGuire.
Ethics
New advances in the detection of bias in face recognition algorithms
A team from the Computer Vision Center (CVC) and the University of Barcelona has published the results of a study that evaluates the accuracy and bias in gender and skin color of automatic face recognition algorithms tested with real world data.
Challenges for Responsible AI Practitioners and the Importance of Solidarity
Recent years have seen an explosion in the study of responsible artificial intelligence (AI), with more resources than ever offering guidelines for mitigating this technology’s harms and equitably distributing its benefits.
The new weapon in the fight against biased algorithms: Bug bounties
When it comes to detecting bias in algorithms, researchers are trying to learn from the information security field – and particularly, from the bug bounty-hunting hackers who comb through software code to identify potential security vulnerabilities.
Robotics
Deep Learning in Self-Driving Cars
Deep Learning has taken over the major subfields of autonomous driving. In this article, I’d like to show you how Deep Learning is used, and where exactly.
Cybersecurity
Dr. Roman Yampolskiy on the growing threats of AI, potential solutions and the future
Over the years, artificial intelligence has become a mainstream technology and can be seen everywhere around us. There’s no denying that it has made life simpler, but on the other hand, AI has also brought to the fore several security concerns.