Special Edition - Scaling dataset labeling with quality and speed
Getting quality labeled data, and getting it fast, is often the obstacle to getting good results from experimental models. In order to stay agile, research teams and startups alike need access to the best training data possible in the shortest amount of time.
When lumber prices rose drastically during the Covid-19 pandemic, measuring the weight, dimensions, and quality of lumber became more important than ever before. Measuring, or scaling lumber has historically been done manually. It’s a dangerous process, requiring individuals to work in environments where logs are piled high, people and heavy machinery work in close proximity. TimberEye provides a mobile application that leverages the latest in computer vision and LiDAR mapping technology to enable lumber suppliers as well as buyers to categorize and scale logs up to 20x faster, more safely and consistently, and with better accuracy. Typical human operators will differ from verified measurements by 1.2 - 1.5cm, but the TimberEye app delivered highly accurate measurements with an average difference to verified manual measurements of just 0.3cm.
To continue to enhance the app’s log scaling capabilities, the TimberEye team wanted to experiment with an instance segmentation model. But semantically segmenting images was a laborious and time-consuming process that delayed experimentation.
Scott Gregg, CEO and Founder of TimberEye said that they kept kicking the segmentation work to the back burner, almost abandoning it. But, three days after kicking off the project with Rapid, they had all the data they needed in perfect shape.
Scale was founded to solve the challenge of scaling data labeling pipelines to production-level volumes.
Sponsor
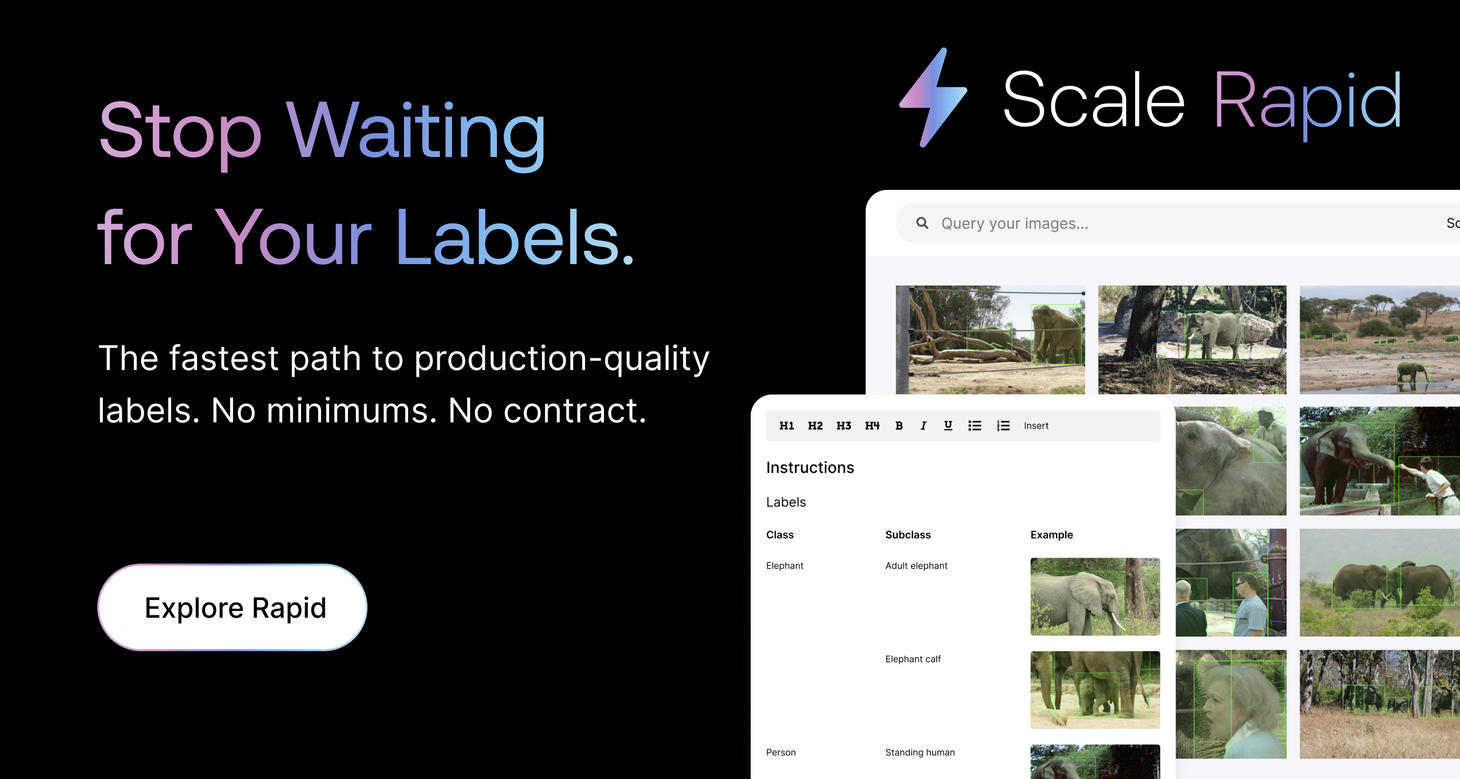
Stop Waiting For Your Labels
Scale Rapid is a new product that enables machine learning engineers and researchers to receive high-quality labels and instruction feedback in as little as one hour.
With Scale Rapid you can:
- Experiment quickly by setting up labeling projects in minutes and receiving initial datasets within hours.
- Iterate over potential edge cases and instructions by getting fast and real-time feedback on labeling instructions and ensure high-quality annotations.
- Scale to production-level pipelines in days with precision quality control. No need for lengthy contract negotiations, purchase order, or committed spend.
In The News
TimberEye. Enhancing Log Scaling and Inventory Management with Scale Rapid
Faster, safer, and more accurate log scaling. TimberEye provides a mobile application that leverages the latest in computer vision and LiDAR mapping technology to enable lumber suppliers as well as buyers to categorize and scale logs up to 20x faster, more safely and consistently, and with better accuracy.
4 Reasons Why You Need To Outsource Your Data Annotation Project
For a lot of companies, the mere idea of developing a simple AI model could push them to assume they would need millions of dollars to develop it. Oftentimes, they turn out to be true as well. However, every cost that you incur should give you significant returns. That’s the only way you know you’ve invested in something wisely.
Scale AI acquires SiaSearch to give companies the same data engine superpowers that Tesla has
Scale AI has acquired a small startup that could help expand its reach in Europe and speed up development of its newest product. The startup, which is already working with automakers like Volkswagen and Porsche, is able to automatically index and structure the raw sensor data collected by fleets of vehicles.
Applied use cases
Improving Reliability of Solar Power with Data Annotation
The ever increasing efficiency of solar panel technology, combined with improvements in manufacturing, installation, and maintenance mean that this vital renewable power resource is set to become a major component in our energy infrastructure.
SimNet In the Home
We assembled a team of researchers from UC-Berkeley, Google, Amazon and MIT, to ask the question: Can we drastically reduce the cost of data annotation? In response, we have developed SimNet, a low-quality procedural simulator for tackling 3D perception in the wild.
Healthcare
GA4GH: International policies and standards for data sharing across genomic research and healthcare: Cell Genomics
The Global Alliance for Genomics and Health (GA4GH) aims to accelerate biomedical advances by enabling the responsible sharing of clinical and genomic data through both harmonized data aggregation and federated approaches.
AI Helps Humans Annotate Biological Data in Real-Time
A new study published in npj Digital Medicine demonstrates how Human-Augmenting Labeling System (HALS) can reduce the manual work of labeling data by over 90 percent and increase the quality of biological data annotation.
Computer vision
Game Changers: Nature Methods
In this blog post, Rita Strack, Handling Editor for Deep learning enables fast and dense single-molecule localization with high accuracy at Nature Methods, describes how deep learning is used to improve super-resolution microscopy, and explains why the journal plays a part in improving laboratory techniques and methods.
Dense Unsupervised Learning for Video Segmentation
This repository contains the official implementation of our paper: Dense Unsupervised Learning for Video Segmentation. Nikita Araslanov, Simone Schaub-Mayer and Stefan Roth. To appear at NeurIPS*2021
Natural language processing
Continuous Learning in NMT using Bilingual Dictionaries
With a large amount of parallel data, we can achieve impressive translation quality with neural machine translation (NMT). The challenge is how to enable NMT to adapt well to new knowledge that is not covered in the training data.
IBM to Add New Natural Language Processing Enhancements to Watson Discovery
RThese planned updates are designed to help business users in industries such as financial services, insurance and legal services enhance customer care and accelerate business processes by uncovering insights and synthesizing information from complex documents.