Operational AI: Achieving Next-Level Value From AI
Technology research firm Gartner has estimated that as much as 85% of all AI and ML projects fail to produce a return for the business. One major reason that organizations struggle to achieve value from AI projects is that, after having invested in data science to create innovative ML models, they fail to adopt the processes, team, and tools to effectively put models into a production environment where they can deliver value.
To avoid this trap and achieve greater value from AI, businesses must stop thinking of models as an end on their own. A model is just a way to transform data written in the form of a function — similar to software. Making the transition from “artisanal” data science to “industrial” ML at scale requires organizations to adopt an Operational AI mindset along these main dimensions:
- People: An ML Platform team responsible for managing the tooling and process for operationalizing models.
- Process: Standardized processes and best practices for putting models into production.
- Technology: An Operational AI platform that specifically supports the requirements for operationalizing, managing and monitoring models in production.
By adopting a software mindset around ML and putting in place the team, processes and tools to safely and efficiently deploy ML models, businesses can significantly increase AI velocity, reduce MLOps cost of ownership, improve data science productivity, and deliver greater value from their AI innovations.
Sponsor
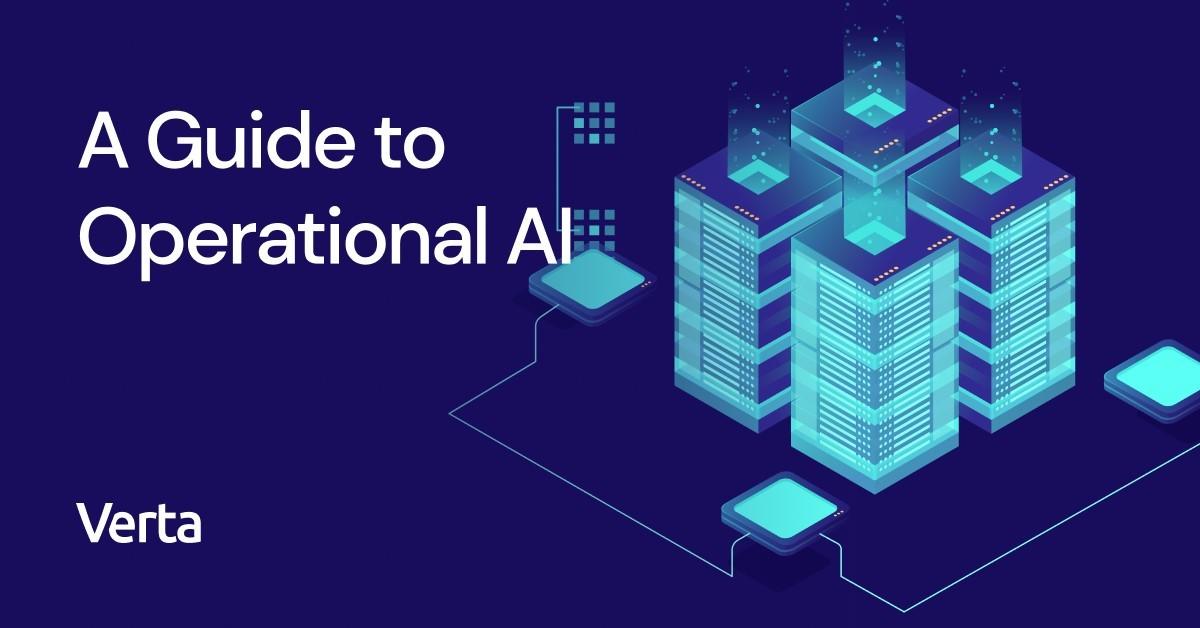
Get Your Guide to Operational AI
What does it take to become an AI-forward organization? Read the Guide to Operational AI and learn how to adopt an Operational AI mindset, including: A roadmap for Operational AI, the people & technology you need, and a reference architecture for AI.
Operational AI
Achieving Next-Level Value From AI By Focusing On The Operational Side Of Machine Learning
A Forbes article highlights the importance of focusing on the operational side of machine learning to achieve the next level of value from AI.
AIOps Market to exceed $38 Bn by 2032
According to Global Market Insights, the AIOps market is set to exceed $38bn by 2032.
AI's New Role In Medicine, Finance And Other Industries
Upstream Works is said to be blazing trails in a competitive industry with its cloud-based solutions for customer service and contact centers.
Operational AI & Security
Understanding AIOps and Its Impact on Cybersecurity Management
AITHority covers the topic of AIOps and its impact on cybersecurity management, explaining the importance of integrating AI into IT operations.
How Lumeo Simplifies Video Analytics With A Low/No Code Vision AI Platform
Forbes introduces Lumeo, a low/no-code vision AI platform that simplifies video analytics.
Use of AI and Machine Learning in Detecting and Preventing Network Security Breaches
The use of AI and machine learning in detecting and preventing network security breaches is highlighted in a news article by Big News Network.
Operational AI & Industries
AI May Actually Help Humanize Financial Services
Joe McKendrick from Forbes argues that AI may actually help humanize financial services.
Lucy 4 is Moving Ahead with Generative AI for Knowledge Management
Artificial Intelligence News covers the release of Lucy 4, a generative AI tool for knowledge management.
Here's How AI can Benefit the Retail Sector
The World Economic Forum discusses how AI can benefit the retail sector, particularly in areas such as personalized shopping experiences and supply chain optimization.
One of the Next Big AI Disruptions could be in the Music Industry
TechXplore reports on the big impact AI is having in the field of text-to-audio generation.